Friday, March 14, 2025
How a DTC Brand Optimized Seasonal Ad Spend & Unlocked Hidden Opportunities with Maxma
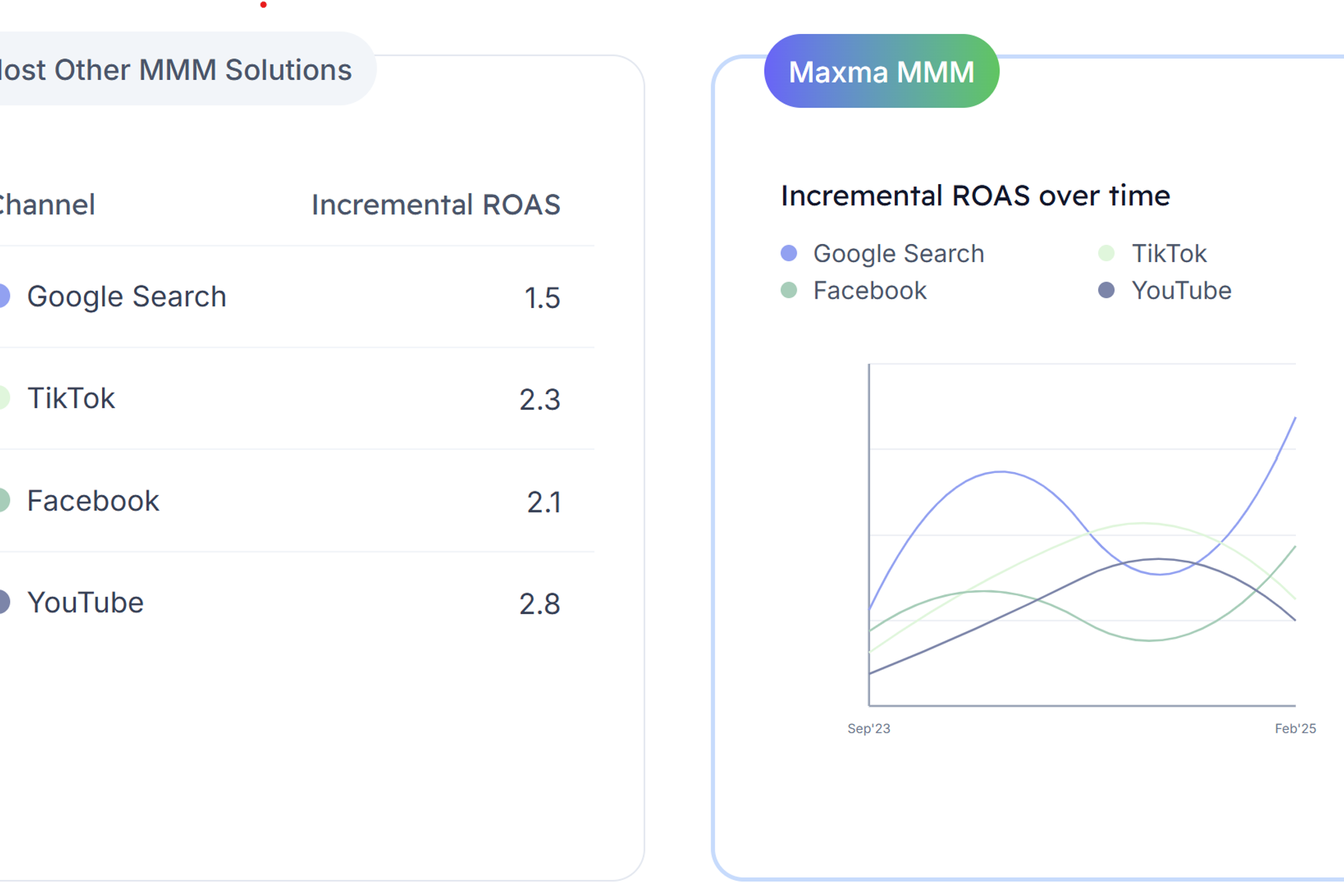
The Brand
A direct-to-consumer (DTC) company with nearly $20M in annual advertising spend. The marketing team is highly experimental, running campaigns across platforms such as TikTok, Pinterest, as well as offline channels like podcast and influencer marketing beyond the biggest channels.
The Challenge
With a highly seasonal business, over 60% of revenue and ad spend is concentrated around a few key holidays. Their media mix optimization challenge was not only about allocating budgets across channels and tactics but also about determining the optimal balance between peak and off-peak seasons.
Additionally, the team faced measurement gaps:
- Their existing tools struggled to measure offline channels and influencer marketing.
- They know their primary attribution tool, which is the platform reportings and google-analytics last touch attributions can be biased, they want to understand their true performance from the lens of incrementality-based measurement.
The Maxma Solution
To address these challenges, the brand adopted Maxma’s Ongoing Marketing Mix Modeling (MMM) to measure the incremental impact of their entire paid advertising portfolio, including offline channels. This provided them with a continuous view of their incrementality throughout the year, allowing them to compare and optimize mix across channels, tactics, and time.
Key Findings
Seasonal Business and Volatile Performance
With 60%+ of their budget concentrated in peak seasons, performance also followed a highly seasonal pattern. During their peak seasons where they drastically boosted spend across channels and tactics, some channels and tactics scaled well, maintaining strong efficiency at higher budgets, while others experienced a sharp rise in cost per incremental new customer during peak times suggesting strong sign of saturation.
We also found even the best-performing channels saw post-peak weaknesses, with declined efficiency for an extended period of time. The team could more aggressively shift the post-peak budget to places with higher yield.
High Potential in Offline Channel
We were able to measure all the offline channels for the client. We identified an offline channel, purchased at a much lower CPM than most digital channels, showing promising results. When measuring pure effectiveness using incremental conversions per thousand impressions, this channel performed only slightly below the brand’s digital top-of-funnel channels. However, because of its substantially lower cost, its overall efficiency and ROAS were much higher.
While these results were promising, measurement limitations existed for the offline channels. For digital channels, we leveraged large-scale geolocation data—1000x the volume of traditional MMM inputs—to achieve a robust and accurate measurement. However, for offline channels, the lack of granular geo-level data reduced confidence in the results. We were transparent with the client about these limitations, framing the goal of offline channel measurement as moving from complete uncertainty to directional evidence, rather than providing high-confidence conclusions.
Incrementality v.s. Platform Attribution
We were able to measure and compare incrementality performance across different campaign tactics - awareness (maximizing reach or web visits), prospecting (broad targeting maximizing conversions), and retargeting - across multiple social media platforms.
Awareness campaigns, which showed minimal conversions in platform, actually drove significant incremental new customers at strong efficiency levels for some channels in the MMM. On the other hand, some retargeting tactics, despite showing high conversions in-platform, had much lower true incremental impact when measured through MMM.
In terms of prospecting, we observed that even when platforms provided automated audience targeting based on conversion signals, the actual incrementality performance varied significantly. This indicated that the platform algorithm capability gap may exist and they do not optimize equally across all advertisers.
From Findings to Action
An Incrementality Testing Roadmap Guided by Scaled MMM Insights
From the client’s own words: “The insights you provided really gave us a lot to chew on and are inspiring ideas for shifting our spend and for new experiments we can be running in the coming months. As we've dug in more, we've especially found it valuable to get this at the tactic level.”
This client has been leveraging Incrementality Testing for a while before adopting MMM. But their tests were often sporadic and reactive. Maxma MMM scanned their entire portfolio, uncovering hypotheses based on incrementality evidence from MMM which guided more strategic and high-impact testing. Instead of blindly testing different areas, they could now prioritize experiments based on MMM insights, maximizing learning while minimizing testing cost.
However, further Incrementality Testing to validate MMM insights is not always necessary. Whether additional testing is needed depends on a brand’s measurement culture, risk tolerance, and how big the bet the decision impacts.
Capturing the “value trough”
The client was excited about the promising results from the offline channel, as it represented a high-potential area for scaling. However, due to data limitations, they preferred additional validation before making a significant investment.
Since the channel did not allow geo-level targeting for a standard Incrementality Test, we recommended running short bursts of spend that misaligned with seasonality and other major channels to create more variation in the data, allowing for better measurement with another round of MMM. The client immediately implemented this test, and as of writing, the results are still in progress.
Validating & Calibrating MMM with Incrementality Testing
The client had previously run Incrementality Tests, which we used to validate MMM accuracy. Because advertising performance is always evolving, we expect MMM and Incrementality Testing results to align only when measuring the same campaigns in the same timeframe.
Where we lacked Incrementality Tests for the exact timeframe, we compared MMM results with past Incrementality Tests from slightly different periods. The differences between MMM and Incrementality Test results were within 20%, whereas platform-reported metrics were about 2x inflated.
Broader Implications
Mix optimization is not just about channels and tactics - but timing
Brands with highly seasonal businesses can experience vastly different incrementality during various times of the year, often leading to overspending or understanding during different periods. Optimization marketing mix isn’t just about channels and tactics—timing matters just as much.
Diverse ad portfolio and identifying the “Value Trough”
By leveraging a diverse portfolio of channels, including those that are harder to measure, this brand was able to discover undervalued opportunities. As media costs continue to rise across platforms like Google and Meta, the most savvy advertisers seek out “value troughs” in alternative channels.
However, without independent measurement, it’s difficult to compare performance fairly across platforms. A capable third-party measurement solution like Maxma MMM ensures that all channels are evaluated on a level playing field.
How MMM and Incrementality Testing can work together
This case study also highlights how MMM and Incrementality Testing work best together. MMM provides high-confidence insights at scale, while Incrementality Testing selectively validates big bets. In turn, Incrementality Test results can be used to fine-tune MMM for even greater accuracy.